Custom Healthcare Software Development: 2020 Approach Vs. 2024 Approach
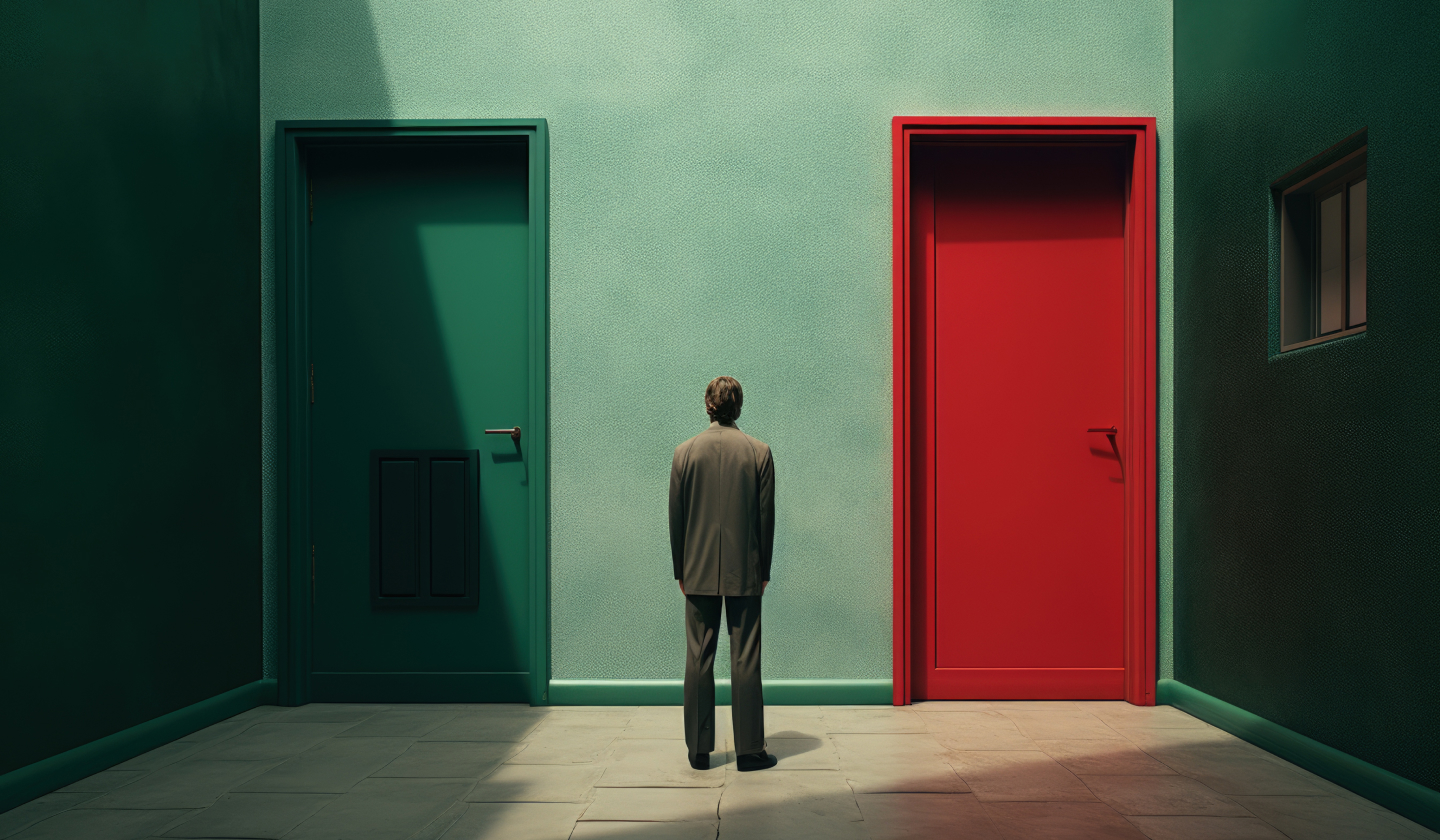
Custom Healthcare Software Development: 2020 Vs. 2024 Approach
- Traditional, document-centric approach.
- Primarily on requirements gathering through interviews, workshops, and documentation.
- Limited use of data analytics, user journey mapping, and prototyping tools.
- Static project scope creation.
- Agile, data-driven, and user-centric approach with rapid prototyping and iterative development.
- Deeper user understanding through data analysis, user journey mapping, and rapid prototyping.
- Leverage AI-powered tools for user persona development, sentiment analysis, and journey mapping.
- Dynamic and data-driven product vision document
- Monolithic architectures were common.
- On-premise deployments were still prevalent.
- The focus was often on internal functionality, with APIs as an afterthought.
- Containerization (Docker, Kubernetes) was gaining traction.
- Microservices architectures dominate.
- Cloud adoption (public, private, hybrid) is widespread.
- API-first design is crucial now.
- Serverless computing, AI/ML integration, and IoT integration are transforming architectures.
Check Out Our Software Architecture Engineering Expertise
- Manual testing methodologies.
- Limited automation, mostly for regression testing.
- Testing is performed in isolated environments, leading to longer testing cycles.
- Limited use of AI/ML for testing purposes.
- Shift towards automated testing.
- Adoption of AI-driven testing tools.
- Integration of testing into DevOps and Agile methodologies.
- Utilization of cloud-based testing platforms for scalability and flexibility.
- UX focused on basic functionality and navigation.
- Static interfaces, limited data integration, focus on desktop or web.
- Patient portals with basic functions and limited user research.
- Limited use of interactive elements and animations in UI.
- UX emphasizes personalization and intuitive workflows.
- Dynamic interfaces, AI-powered personalization, multi-device accessibility.
- Empathetic design, data-driven personalization, gamification for engagement.
- Voice interfaces, micro-interactions, AR/VR integration, intuitive navigation.
- Basic optimization techniques like code profiling, caching, and database indexing.
- Primarily on-premise infrastructure, limited focus on cloud scalability.
- Focus on optimizing code and infrastructure for baseline performance.
- Reactive performance testing, limited scalability in infrastructure.
- AI/ML-driven performance analysis, proactive identification of bottlenecks.
- Leveraging cloud infrastructure for dynamic scaling and microservices architecture.
- Focus on UX and continuous improvement with AI/ML-driven optimization.
- Optimize website code with an online Minifier to improve its performance.
- Proactive performance analysis with AI, cloud-based infrastructure for elastic scaling.
- Limited scalability due to monolithic architecture.
- Relational databases that struggled with unstructured data like medical images.
- Required manual intervention to add resources.
- Costly and had limitations in handling sudden spikes in traffic or data volume.
- Custom healthcare software development emphasizes microservices architecture.
- Efficiently handle large volumes of unstructured data with NoSQL databases.
- Leverages cloud platforms like AWS or Azure for elastic scaling.
- Automated tools to manage resource allocation based on predefined rules.
- Focus on legacy security protocols
- On-premise data storage is common, increasing the attack surface.
- Focus on known vulnerabilities, leaving blind spots for new threats.
- Firewalls and intrusion detection systems formed the main defense.
- Shift to stronger protocols and post-quantum cryptography readiness.
- Cloud-based storage with robust provider security features is preferred.
- DevSecOps that implements security into the development process, not bolted on later.
- Encryption throughout the data lifecycle (at-rest, in-transit, in-use).
- Relied heavily on traditional version control systems SVN.
- Limited branching options
- Change tracking and compliance checks primarily manual
- Manual integration with compliance tools.
- Increased use of Git for distributed version control
- Advanced branching strategies and tools (e.g., Gitlab, Github) for complex workflows.
- Automated tracking and compliance
- CI/CD pipelines integrate version control with automated testing and security scans.
- CI/CD pipelines were manually configured and maintained.
- Limited automation resulted in slower release cycles and error-prone.
- Testing and deployment processes were typically separate.
- Legacy build tools, limited use of cloud platforms, static infrastructure.
- CI/CD pipelines are fully automated and orchestrated using advanced DevOps tools and platforms.
- Automated testing is seamlessly integrated into the CI/CD pipeline.
- IaC principles are employed for defining and managing infrastructure resources.
- Utilization of Docker and Kubernetes for consistent deployment across different environments.
Check Out Our Managed DevOps Services
- Primarily paper-based documentation or static PDFs.
- Limited collaboration due to manual updates and version control is cumbersome.
- Emphasis on detailed compliance checklists and reports.
- Restricted access within healthcare organizations.
- Cloud-based platforms facilitate real-time access and updates.
- Tools enabling team-based document creation and modification.
- Automated compliance with built-in checks and reports ensures regulatory adherence.
- Secure access for authorized users across organizations
- Maintenance focused on fixing bugs and issues after they arose.
- Updates and patches are applied manually, leading to potential delays and downtime.
- Limited monitoring due to relying on user reports for issue identification.
- Manual testing and deployment, increasing maintenance costs and human error.
- Predictive analytics are used to anticipate and prevent issues.
- Tools and scripts for automated updates, patches, and deployments.
- Continuous monitoring with AI-driven algorithms.
- Automated testing and deployment pipelines for faster, more reliable updates.
- Error handling in custom healthcare software development typically relies on basic try-catch blocks and manual error logging.
- Debugging and troubleshooting were time-consuming.
- Manual error recovery processes with limited automation.
- Reactive error handling. Catching and logging errors to identify them later.
- Advanced error handling techniques, leveraging automated monitoring and proactive error detection mechanisms.
- AI-driven anomaly detection and predictive analysis.
- Leverages self-healing mechanisms and API resilience techniques for automatic recovery.
- Proactive error handling. Predicting and preventing errors before they occur.
- Relied on experienced developers to meticulously check code.
- Basic static analysis tools for initial checks, but deeper scrutiny is done manually.
- Reviews are often restricted to smaller teams or individual developers.
- Detailed comments and reports outlining findings.
- Increased use of automated tools for code quality, security, and performance checks.
- AI-powered assistance to identify complex issues and automate repetitive tasks.
- Collaborative code review and real-time feedback within larger teams.
- Metrics and automated reports provide clearer insights and track progress over time.
- Focus on readability and maintainability
- Ensuring individual code blocks function as intended.
- Teams rely on human analysis for code quality.
- Limited use of static analysis tools, primarily for basic checks like syntax and potential bugs.
- Enhanced focus on code quality assurance and improvement
- Streamlining the testing process and improving coverage.
- Greater emphasis on writing testable code.
- Integration of automated code analysis tools and AI-driven code review systems.
Explore Our Software Product Development Offerings
- Prevalent use of tools for manual dependency installation and updates.
- High risk of version conflicts leading to compatibility issues and bugs.
- Dependencies specific to projects, hinder code reuse across projects.
- Less focus on dependency security vulnerabilities.
- Increased adoption of containerization tools like Docker and Kubernetes for isolated and controlled environments.
- Dependencies packaged within containers, eliminating version conflicts.
- Utilizing microservices architecture, promoting modularity and code reuse.
- Integration of automated dependency vulnerability scanning and patching tools.
- Primarily focused on identifying issues after they occurred.
- Limited log data capture, mostly basic logs like errors and user actions.
- Relied on manual inspection of logs for root cause analysis.
- Logs are stored on local servers, limiting scalability and accessibility.
- Utilizes real-time analytics to predict and prevent potential issues.
- Captures detailed logs including system metrics, API calls, and user behavior.
- Utilizes machine learning for automated issue identification and root cause analysis.
- Cloud-based storage that enables scalable and centralized log management.
- Monolithic architecture was prevalent.
- Limited scalability and flexibility due to the dependency on a single codebase.
- Challenges in updating or adding new features without disrupting the entire system.
- Traditional relational databases like SQL were commonly used for data storage.
- Microservices architecture is widely adopted.
- Enables scalability and flexibility by allowing each service to be developed, deployed, and updated independently.
- Simplifies integration of new features and technologies without affecting the entire system.
- Adopts NoSQL databases for efficient management of diverse healthcare data at scale.
- Relied heavily on established unit testing frameworks like JUnit, NUnit, and XUnit.
- Testing primarily focused on individual units of code in isolation.
- Limited support for integration testing and end-to-end testing within the same framework.
- Emphasis on manual testing for system-level validation.
- Adoption of more advanced unit testing frameworks such as Jest, Mocha, and Cypress.
- Shift towards Test-Driven Development (TDD) and Behavior-Driven Development (BDD) methodologies.
- Integration of unit tests with CI/CD pipelines for automated validation.
- Increased use of containerization for environment consistency in testing.
- Relational databases like SQL were predominantly used for custom healthcare software development.
- Emphasis on normalization to reduce redundancy and maintain data integrity.
- Performance optimization through indexing and query optimization techniques.
- Limited scalability due to the rigid structure of relational databases.
- Adoption of NoSQL databases for more flexibility in handling diverse and unstructured healthcare data.
- Integration of graph databases for complex relationships in healthcare networks.
- Emphasis on distributed databases for scalability and fault tolerance.
- Utilization of ML algorithms for dynamic database optimization and predictive analytics.
- Prevalent use of RESTful APIs due to their simplicity and widespread adoption.
- FHIR was an emerging standard but adoption was not yet widespread.
- Security primarily focused on authorization.
- Some legacy systems still rely on SOAP APIs for data exchange.
- FHIR has become the dominant standard for healthcare data exchange due to its flexibility and interoperability.
- API gateways are used for complex management and security.
- Growing compliance concerns like GDPR and HIPAA lead to stronger data privacy measures.
- Deeper integration with serverless computing for scalability and cost-effectiveness.
- DevOps relied on manual intervention for CI/CD processes.
- Proactive monitoring of applications and infrastructure for performance issues and errors.
- Automated tasks like testing, deployment, and infrastructure provisioning for efficiency and reduced human error.
- DevOps emphasizes a manual approach to resource management and scaling decisions.
- AIOps utilizes ML algorithms during CI/CD processes to identify patterns, anomalies, and potential issues.
- AI analyzes performance data to identify bottlenecks and suggest optimizations.
- AI predicts potential hardware failures based on sensor data.
- AIOps leverages ML algorithms to analyze real-time data and predict resource demands.