6 reasons your models struggle—and how we solve them
- Model performance degradation over time
- Inability to detect subtle drifts in predictions
- Frequent retraining without meaningful performance gains
- Overfitting or underfitting issues post-deployment
- Inconsistent results across environments
- Difficulty replicating past model behaviors for audits
- Input data distributions changing over time
- Emerging patterns that models weren’t trained on
- Lack of alerts when drift thresholds are crossed
- Challenges in differentiating noise vs actual drift
- Concept drift due to evolving business dynamics
- Missing real-world context in data tracking
- No visibility into live model performance
- Delayed identification of performance drop-offs
- Lack of centralized dashboards for monitoring
- Fragmented logs and tracking across systemss
- Limited ability to compare versions over time
- Manual and error-prone performance reporting
- Hidden model biases affecting critical decisions
- Regulatory compliance blind spots
- Lack of explainability in model decisions
- Difficulty proving fairness in model outcomes
- No audit trail of prediction reasoning
- Challenges in aligning with ethical AI standards
- Models not optimized for production-scale inference
- Performance bottlenecks under high traffic
- Infrastructure costs rising with low returns
- Difficulty scaling monitoring across multiple models
- Complex CI/CD for ML pipelines
- Latency issues affecting real-time applications
- Difficulty linking model outputs to business KPIs
- Underperforming models in customer-facing roles
- Low stakeholder confidence due to model inconsistency
- Lack of alignment between model goals and business goals
- Overdependence on manual tuning
- Ineffective prioritization of high-impact model optimizations
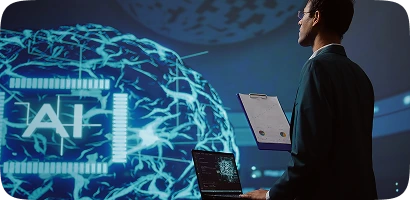
What We Do: Monitor model performance in real-world settings.
How We Do: Automate checks for accuracy, drift, and key metrics.
The Result You Get: Reliable models that adapt to data changes.
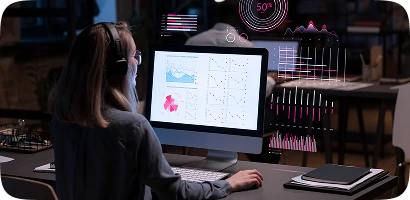
What We Do: Bring all model insights into one dashboard.
How We Do: Integrate metrics, alerts, and trends across systems.
The Result You Get: Complete visibility and quicker interventions.
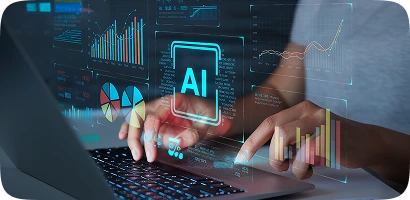
What We Do: Improve model accuracy and efficiency.
How We Do It: Apply retraining, diagnostics, and tuning techniques.
The Result You Get: Optimized models built for real-world impact.
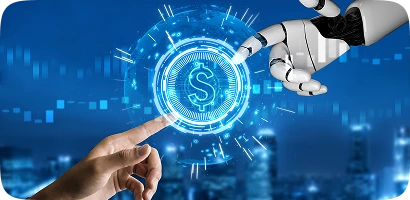
What We Do: Maximize performance without overspending.
How We Do It: Analyze trade-offs between accuracy, speed, and cost.
The Result You Get: Efficient, scalable, and budget-friendly models.
What success looks like with optimized models
Your models continue delivering accurate, relevant outputs even as data shifts. No surprises—just reliable predictions that keep up with the real world.
Proactive monitoring and automated alerts help you detect drift early and act fast, minimizing performance drops and costly business disruptions.
By optimizing both performance and infrastructure usage, you get more value from your models—better decisions, lower costs, and smarter scaling.
Centralized dashboards give your team a clear view of model health and performance, making it easier to track, troubleshoot, and improve outcomes.
In search of ML Monitoring and Performance Optimization partner?
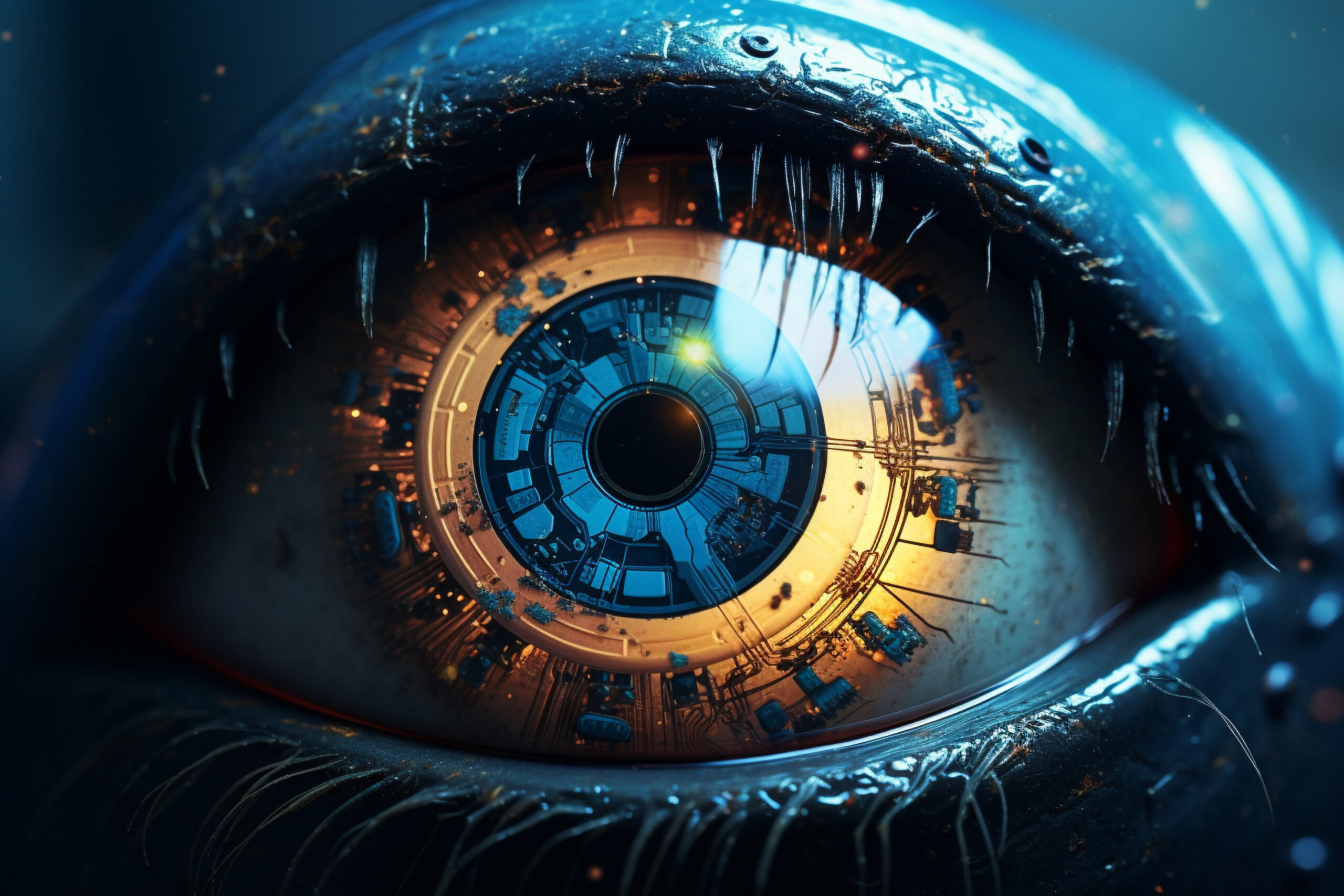
Unlimited
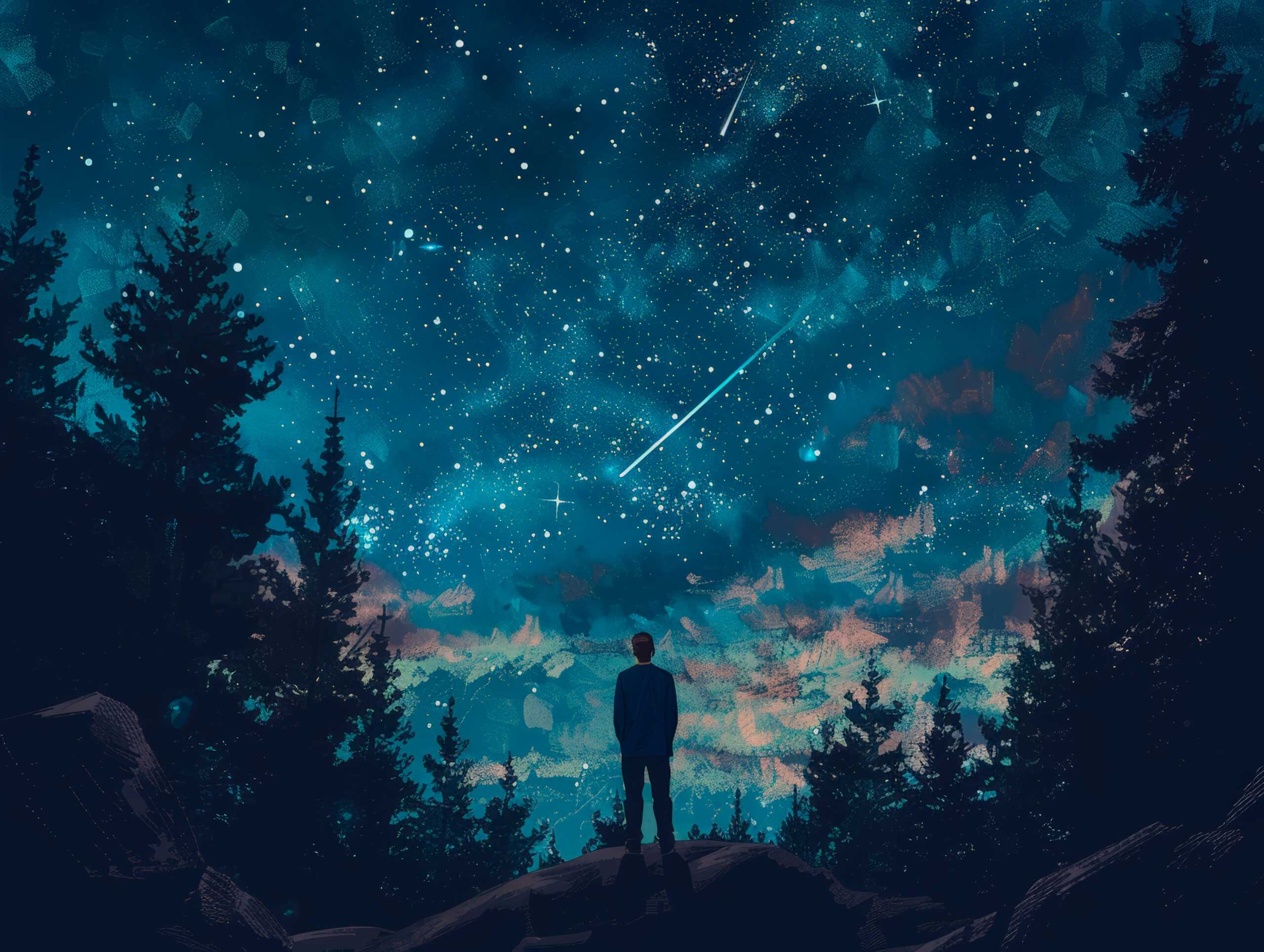
View
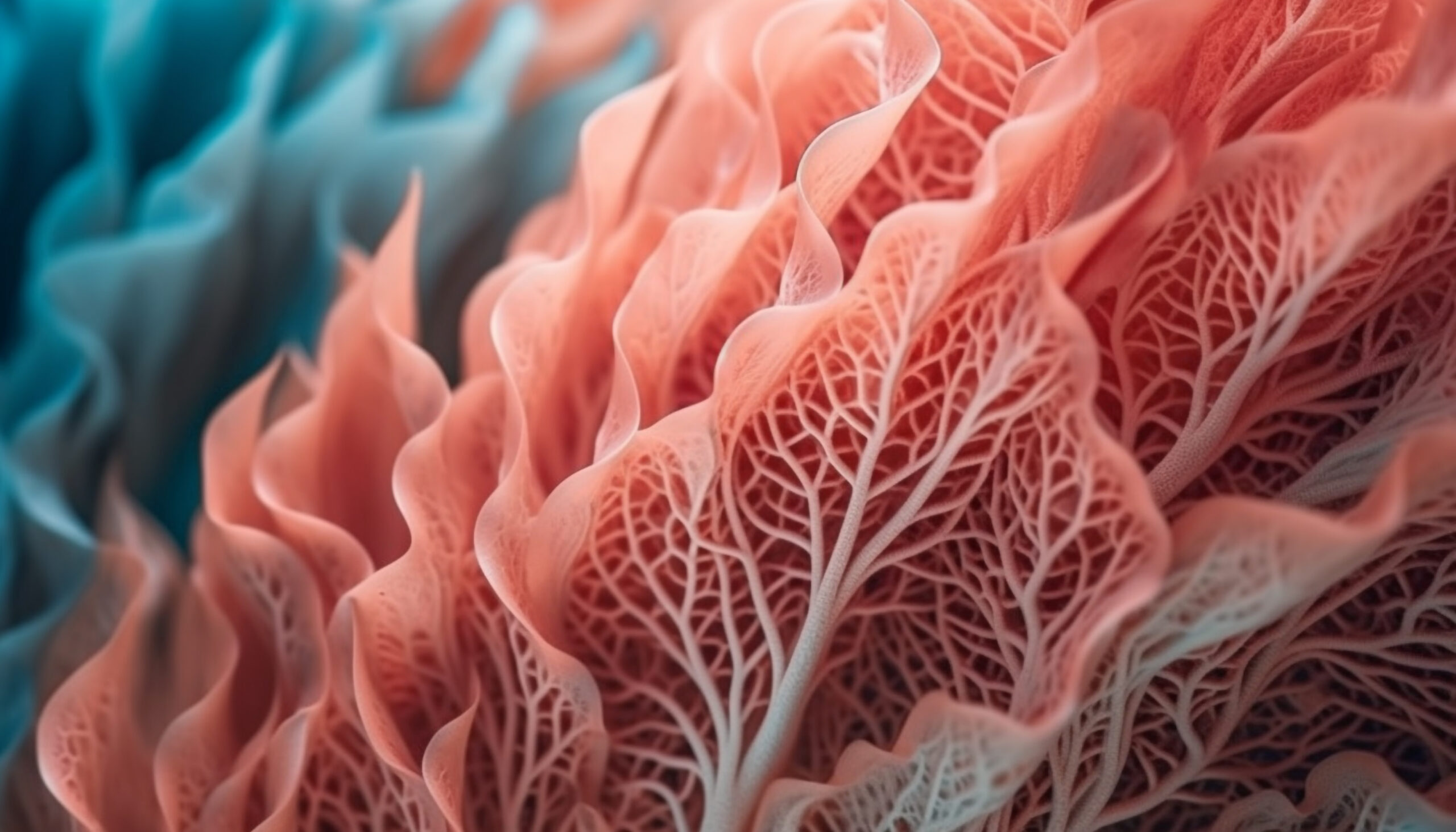
View
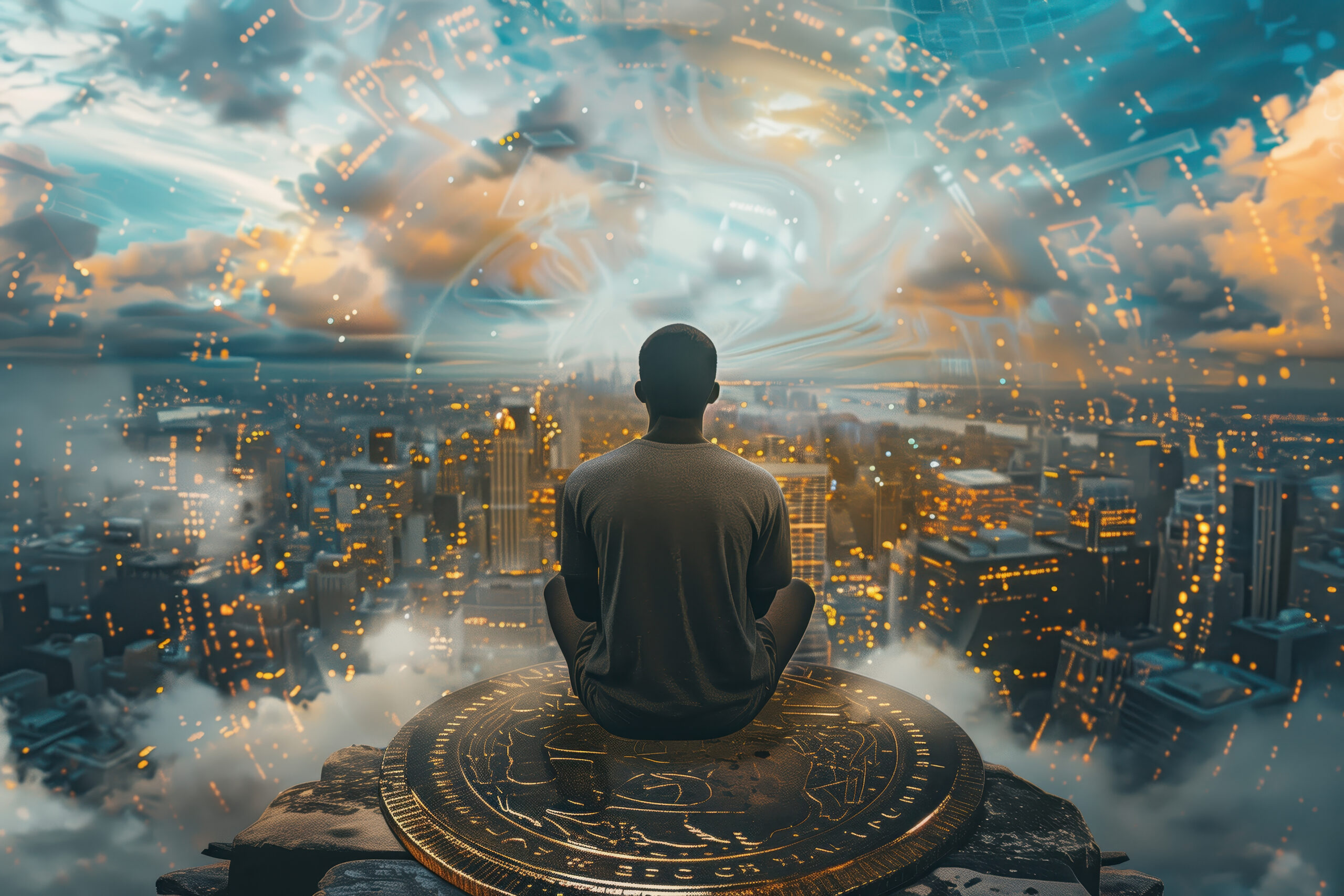
Tactics
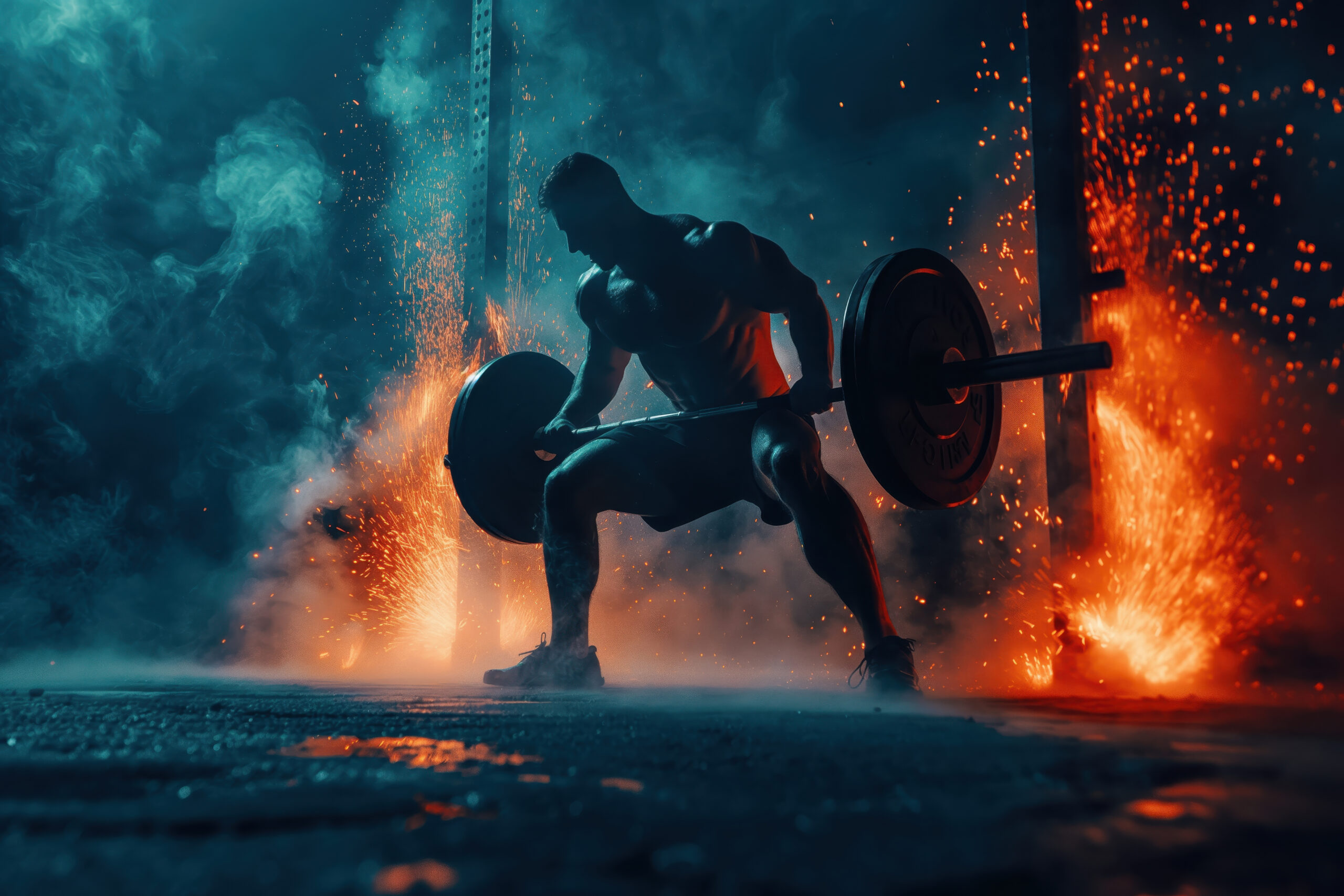
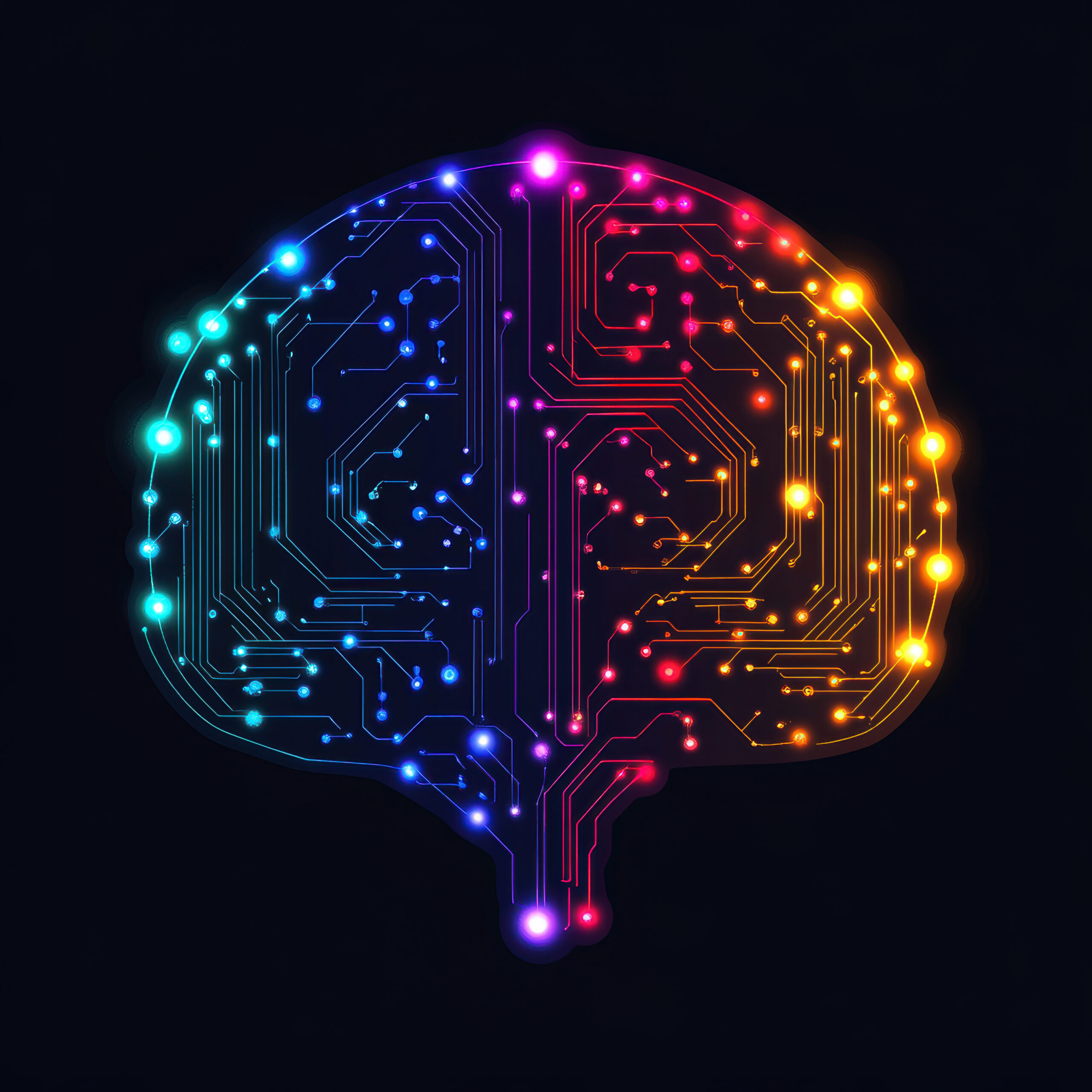
Sense
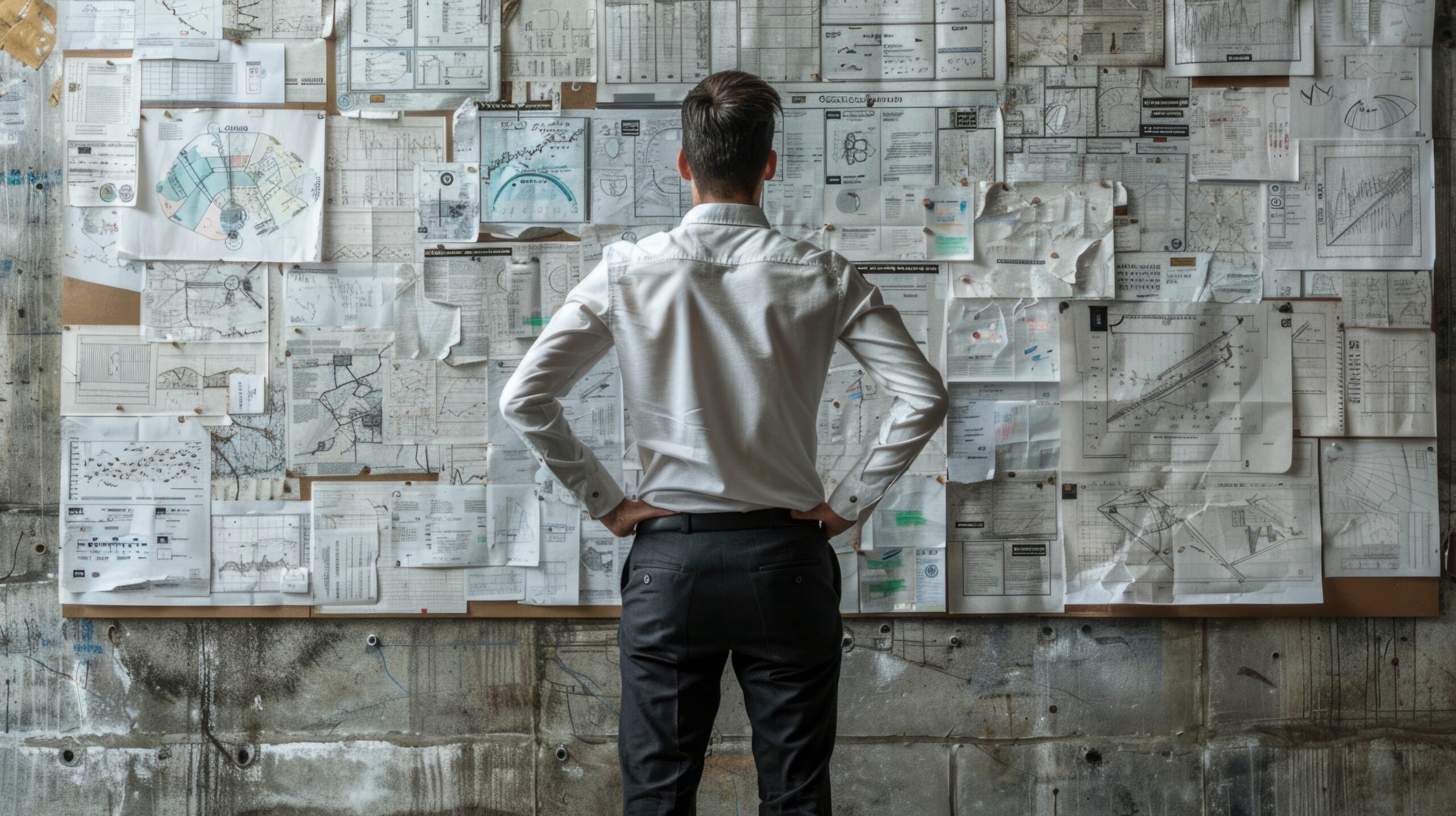
with
Problem
Statement
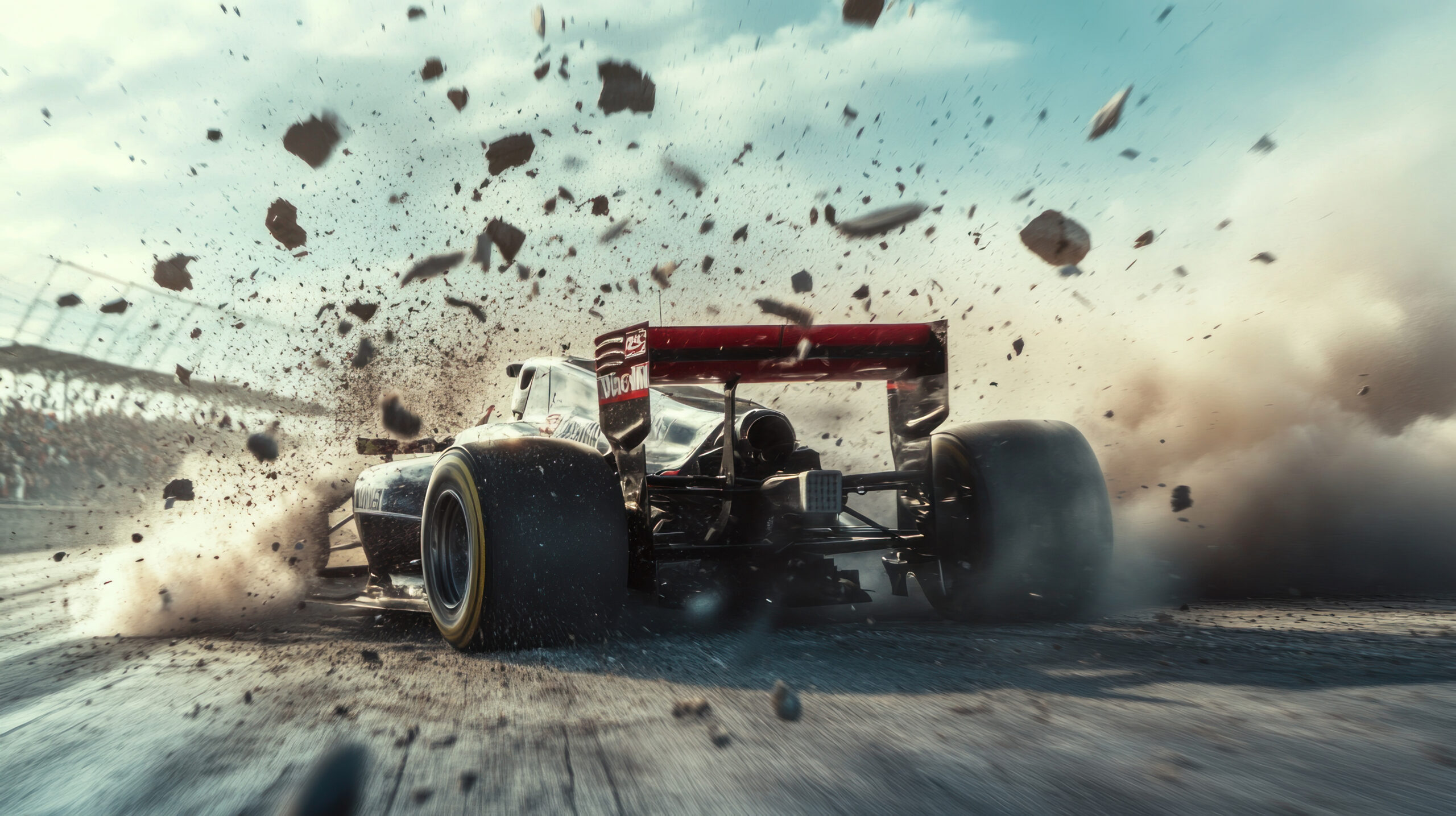
Fast
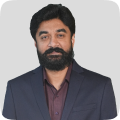
Enabling product owners to stay ahead with strategic AI and ML deployments that maximize performance and impact