Leveraging Talent intelligence in AI-Era Recruitment
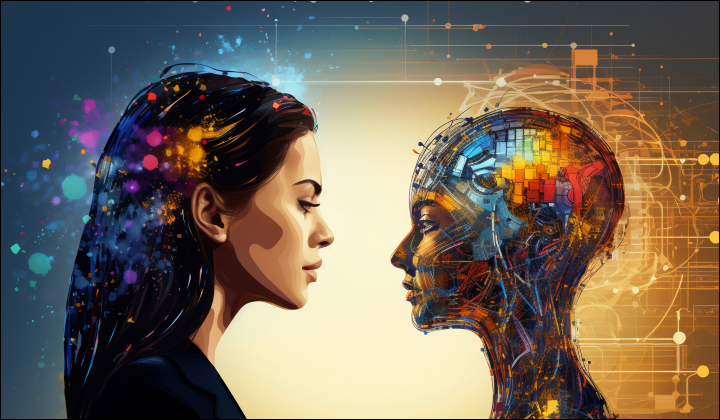
Typically, these HR process optimizations, evolutions, or transformations emanated from two types of circumstances.
1️⃣ One type of scenario involved major HCM technology innovation, often not readily foreseen.
2️⃣ The other related to a change in how the process was conceptualized or thought about in order to garner more compelling business benefits if not sustained competitive advantage.
The latter type of significant progress in an HR process or HCM activity area, along with the various ways HCM Technology enables the corresponding new vision or strategy, often leads to a proliferation of new use cases for innovative technology.
Examples might include
The discipline of Compensation Management evolving into the more holistic Total Rewards Management model, or Vendor Management (around procuring contingent labor) evolving into the discipline of Extended Workforce Management (which includes many more strategic aspects), or Workforce Planning evolving into Strategic Workforce Planning which is much more inter-connected across business functions and stakeholders and continuous in nature.
With that as a backdrop, let’s recognize that the Recruiting or Talent Acquisition domain has witnessed a steady stream of more sophisticated and effective practices replacing former iterations, in other words, the evolution of this domain has occurred as well.
Results such as the ability to attract and retain top, best-fit talent while also managing costs out of the process are now much more attainable, of course with the help of better technology and user experiences.
That said, more impactful strategies and approaches in this arena, as with all the other HR process areas, are usually a direct consequence of having and applying more HCM-related data insights in the flow of work.
What is talent intelligence (TI)?
Talent intelligence refers to the efforts of an organization to collect, analyze, and leverage as a true asset all useful and reliable internal and external workforce, industry, and labor market data.
External ‘TI’ data is often largely about the company’s competition and surrounding industry dynamics and data points. Internal information in the scope of a talent intelligence initiative almost always includes current and future labor and skill needs and corresponding gaps.
Moreover, talent intelligence efforts are usually more successful if external data or industry benchmarks are used within comparisons against an organization’s trends and results.
Just as an AI image generator can create visuals by combining different data inputs to produce a cohesive image, talent intelligence synthesizes various data points to form a clear and actionable picture of workforce dynamics.
Compensation practices or the pricing of jobs and job families is considered foundational benchmark data, but other common data comparisons and trendlines in the TI realm include various staffing ratios, cuts of demographic data, KPI’s like revenue per employee, engagement, retention, and turnover rates and trendlines, relating the same to customer satisfaction levels, internal mobility and promotion rates, sources of new hires, candidate flow activity, trends around workforce size, HR department staffing in relation to workforce size (e.g., 1 HR staff per 100 employees is an oft-cited stat), time to productivity, etc.
‘PA’ encompasses the broader set of data that might relate to performance, engagement, productivity, or attrition. Examining this type of PA data, companies can make decisions regarding talent management, workforce planning, organizational design, and operational improvements among many other important HR / HCM endeavors.
➡️ As workforce, labor market and HCM-related data insights (aka talent intelligence) get increasingly diverse, far-reaching and inter-connected, more opportunities will no doubt arise for businesses investing in both TI and PA.
Of course, recruitment marketing in the AI 🤖 era also involves elevating the level of personalization in crafting the most effective employer value proposition, the ability to infer the presence of skills even if not explicitly highlighted or culture fit based on past job successes and employer reputations, improving employer brand attractiveness by following the prescriptive guidance of what approaches and strategies have worked to-date, and the degree of candidate fit being assessed across more dimensions than ever.
Also, with respect to the sourcing and use of gig workers, contingents and contractors, today’s corresponding set of processes clearly extends further in scope than just procurement, deployment and cost management elements.
⚠️ Other critical activities relative to these non-employees include optimizing their scheduling, onboarding, evaluation and as-needed coaching while still ensuring compliance with all relevant IRS worker classification and management rules.
Finally, it should be noted that whether an organization uses gig workers, contractors, contingents or regular employees, all these workforce segments can help, or hurt, the reputation of a business.
Key technology implications
There’s no question that interoperability sits at the heart of HR digital transformation and TA is no exception. This is because interoperability is all about facilitating the integration of various, often disparate systems and technologies for fluid communication and data sharing.
Naturally, this pertains to the various data insights across the talent intelligence spectrum, as the typical organization is using and working with data from at least 6-8 HR / HCM technology platforms to service both the operational and strategic HCM needs of the HR department, line managers, employees, corporate leadership, government agencies and external partners.
It was formerly, commonly understood that enterprise systems – HCM or non-HCM related that needed to be linked – should communicate data in a shared structural format. This used to be referred to as semantic interoperability, which was often the goal, but now it extends to ensuring that exchanged information has the same meaning for the receiving system as it does for the sending system.
This requires the use of metadata and controlled vocabularies or ontologies so that the data is not just structurally correct but also contextually meaningful!